Data analytics case studies reveal how businesses harness data for informed decisions and growth.
For aspiring data professionals, mastering the case study process will enhance your skills and increase your career prospects.
So, how do you approach a case study?
Use these steps to process a data analytics case study:
Understand the Problem: Grasp the core problem or question addressed in the case study.
Collect Relevant Data: Gather data from diverse sources, ensuring accuracy and completeness.
Apply Analytical Techniques: Use appropriate methods aligned with the problem statement.
Visualize Insights: Utilize visual aids to showcase patterns and key findings.
Derive Actionable Insights: Focus on deriving meaningful actions from the analysis.
This article will give you detailed steps to navigate a case study effectively and understand how it works in real-world situations.
By the end of the article, you will be better equipped to approach a data analytics case study, strengthening your analytical prowess and practical application skills.
Let’s dive in!
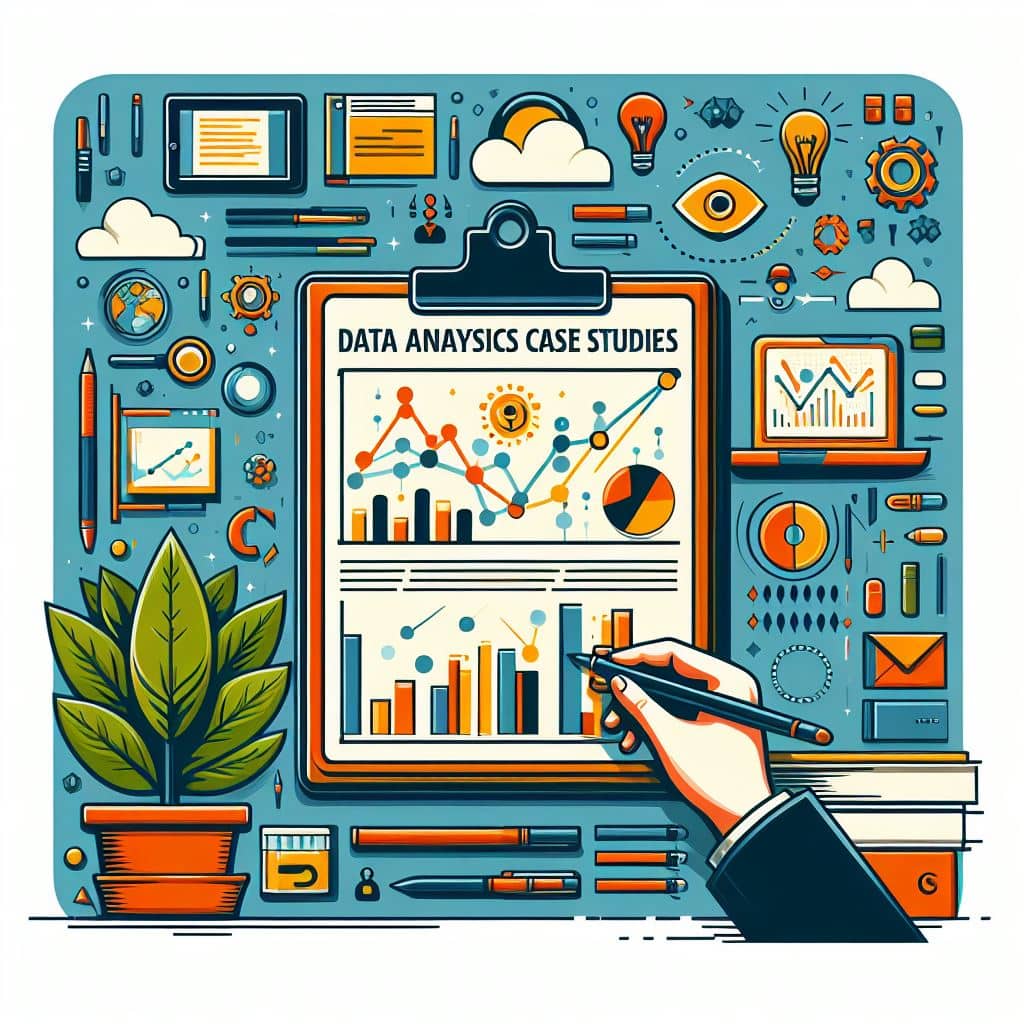
What is a Data Analytics Case Study?
A data analytics case study is a real or hypothetical scenario where analytics techniques are applied to solve a specific problem or explore a particular question.
It’s a practical approach that uses data analytics methods, assisting in deciphering data for meaningful insights. This structured method helps individuals or organizations make sense of data effectively.
Additionally, it’s a way to learn by doing, where there’s no single right or wrong answer in how you analyze the data.
So, what are the components of a case study?
Key Components of a Data Analytics Case Study
A data analytics case study comprises essential elements that structure the analytical journey:
Problem Context: A case study begins with a defined problem or question. It provides the context for the data analysis, setting the stage for exploration and investigation.
Data Collection and Sources: It involves gathering relevant data from various sources, ensuring data accuracy, completeness, and relevance to the problem at hand.
Analysis Techniques: Case studies employ different analytical methods, such as statistical analysis, machine learning algorithms, or visualization tools, to derive meaningful conclusions from the collected data.
Insights and Recommendations: The ultimate goal is to extract actionable insights from the analyzed data, offering recommendations or solutions that address the initial problem or question.
Now that you have a better understanding of what a data analytics case study is, let’s talk about why we need and use them.
Why Case Studies are Integral to Data Analytics
Case studies serve as invaluable tools in the realm of data analytics, offering multifaceted benefits that bolster an analyst’s proficiency and impact:
Real-Life Insights and Skill Enhancement: Examining case studies provides practical, real-life examples that expand knowledge and refine skills. These examples offer insights into diverse scenarios, aiding in a data analyst’s growth and expertise development.
Validation and Refinement of Analyses: Case studies demonstrate the effectiveness of data-driven decisions across industries, providing validation for analytical approaches. They showcase how organizations benefit from data analytics. Also, this helps in refining one’s own methodologies
Showcasing Data Impact on Business Outcomes: These studies show how data analytics directly affects business results, like increasing revenue, reducing costs, or delivering other measurable advantages. Understanding these impacts helps articulate the value of data analytics to stakeholders and decision-makers.
Learning from Successes and Failures: By exploring a case study, analysts glean insights from others’ successes and failures, acquiring new strategies and best practices. This learning experience facilitates professional growth and the adoption of innovative approaches within their own data analytics work.
Including case studies in a data analyst’s toolkit helps gain more knowledge, improve skills, and understand how data analytics affects different industries.
Using these real-life examples boosts confidence and success, guiding analysts to make better and more impactful decisions in their organizations.
But not all case studies are the same.
Let’s talk about the different types.
Types of Data Analytics Case Studies
Data analytics encompasses various approaches tailored to different analytical goals:
Exploratory Case Study: These involve delving into new datasets to uncover hidden patterns and relationships, often without a predefined hypothesis. They aim to gain insights and generate hypotheses for further investigation.
Predictive Case Study: These utilize historical data to forecast future trends, behaviors, or outcomes. By applying predictive models, they help anticipate potential scenarios or developments.
Diagnostic Case Study: This type focuses on understanding the root causes or reasons behind specific events or trends observed in the data. It digs deep into the data to provide explanations for occurrences.
Prescriptive Case Study: This case study goes beyond analytics; it provides actionable recommendations or strategies derived from the analyzed data. They guide decision-making processes by suggesting optimal courses of action based on insights gained.
Each type has a specific role in using data to find important insights, helping in decision-making, and solving problems in various situations.
Regardless of the type of case study you encounter, here are some steps to help you process them.
Roadmap to Handling a Data Analysis Case Study
Embarking on a data analytics case study requires a systematic approach, step-by-step, to derive valuable insights effectively.
Here are the steps to help you through the process:
Step 1: Understanding the Case Study Context: Immerse yourself in the intricacies of the case study. Delve into the industry context, understanding its nuances, challenges, and opportunities.
Identify the central problem or question the study aims to address. Clarify the objectives and expected outcomes, ensuring a clear understanding before diving into data analytics.
Step 2: Data Collection and Validation: Gather data from diverse sources relevant to the case study. Prioritize accuracy, completeness, and reliability during data collection. Conduct thorough validation processes to rectify inconsistencies, ensuring high-quality and trustworthy data for subsequent analysis.
Step 3: Problem Definition and Scope: Define the problem statement precisely. Articulate the objectives and limitations that shape the scope of your analysis. Identify influential variables and constraints, providing a focused framework to guide your exploration.
Step 4: Exploratory Data Analysis (EDA): Leverage exploratory techniques to gain initial insights. Visualize data distributions, patterns, and correlations, fostering a deeper understanding of the dataset. These explorations serve as a foundation for more nuanced analysis.
Step 5: Data Preprocessing and Transformation: Cleanse and preprocess the data to eliminate noise, handle missing values, and ensure consistency. Transform data formats or scales as required, preparing the dataset for further analysis.
Step 6: Data Modeling and Method Selection: Select analytical models aligning with the case study’s problem, employing statistical techniques, machine learning algorithms, or tailored predictive models.
In this phase, it’s important to develop data modeling skills. This helps create visuals of complex systems using organized data, which helps solve business problems more effectively.
Understand key data modeling concepts, utilize essential tools like SQL for database interaction, and practice building models from real-world scenarios.
Furthermore, strengthen data cleaning skills for accurate datasets, and stay updated with industry trends to ensure relevance.
Step 7: Model Evaluation and Refinement: Evaluate the performance of applied models rigorously. Iterate and refine models to enhance accuracy and reliability, ensuring alignment with the objectives and expected outcomes.
Step 8: Deriving Insights and Recommendations: Extract actionable insights from the analyzed data. Develop well-structured recommendations or solutions based on the insights uncovered, addressing the core problem or question effectively.
Step 9: Communicating Results Effectively: Present findings, insights, and recommendations clearly and concisely. Utilize visualizations and storytelling techniques to convey complex information compellingly, ensuring comprehension by stakeholders.
Step 10: Reflection and Iteration: Reflect on the entire analysis process and outcomes. Identify potential improvements and lessons learned. Embrace an iterative approach, refining methodologies for continuous enhancement and future analyses.
This step-by-step roadmap provides a structured framework for thorough and effective handling of a data analytics case study.
Now, after handling data analytics comes a crucial step; presenting the case study.
Presenting Your Data Analytics Case Study
Presenting a data analytics case study is a vital part of the process. When presenting your case study, clarity and organization are paramount.
To achieve this, follow these key steps:
Structuring Your Case Study: Start by outlining relevant and accurate main points. Ensure these points align with the problem addressed and the methodologies used in your analysis.
Crafting a Narrative with Data: Start with a brief overview of the issue, then explain your method and steps, covering data collection, cleaning, stats, and advanced modeling.
Visual Representation for Clarity: Utilize various visual aids—tables, graphs, and charts—to illustrate patterns, trends, and insights. Ensure these visuals are easy to comprehend and seamlessly support your narrative.
Highlighting Key Information: Use bullet points to emphasize essential information, maintaining clarity and allowing the audience to grasp key takeaways effortlessly. Bold key terms or phrases to draw attention and reinforce important points.
Addressing Audience Queries: Anticipate and be ready to answer audience questions regarding methods, assumptions, and results. Demonstrating a profound understanding of your analysis instills confidence in your work.
Integrity and Confidence in Delivery: Maintain a neutral tone and avoid exaggerated claims about findings. Present your case study with integrity, clarity, and confidence to ensure the audience appreciates and comprehends the significance of your work.
By organizing your presentation well, telling a clear story through your analysis, and using visuals wisely, you can effectively share your data analytics case study.
This method helps people understand better, stay engaged, and draw valuable conclusions from your work.
We hope by now, you are feeling very confident processing a case study. But with any process, there are challenges you may encounter.
Key Challenges in Data Analytics Case Studies
A data analytics case study can present various hurdles that necessitate strategic approaches for successful navigation:
Challenge 1: Data Quality and Consistency
Challenge: Inconsistent or poor-quality data can impede analysis, leading to erroneous insights and flawed conclusions.
Solution: Implement rigorous data validation processes, ensuring accuracy, completeness, and reliability. Employ data cleansing techniques to rectify inconsistencies and enhance overall data quality.
Challenge 2: Complexity and Scale of Data
Challenge: Managing vast volumes of data with diverse formats and complexities poses analytical challenges.
Solution: Utilize scalable data processing frameworks and tools capable of handling diverse data types. Implement efficient data storage and retrieval systems to manage large-scale datasets effectively.
Challenge 3: Interpretation and Contextual Understanding
Challenge: Interpreting data without contextual understanding or domain expertise can lead to misinterpretations.
Solution: Collaborate with domain experts to contextualize data and derive relevant insights. Invest in understanding the nuances of the industry or domain under analysis to ensure accurate interpretations.
Challenge 4: Privacy and Ethical Concerns
Challenge: Balancing data access for analysis while respecting privacy and ethical boundaries poses a challenge.
Solution: Implement robust data governance frameworks that prioritize data privacy and ethical considerations. Ensure compliance with regulatory standards and ethical guidelines throughout the analysis process.
Challenge 5: Resource Limitations and Time Constraints
Challenge: Limited resources and time constraints hinder comprehensive analysis and exhaustive data exploration.
Solution: Prioritize key objectives and allocate resources efficiently. Employ agile methodologies to iteratively analyze and derive insights, focusing on the most impactful aspects within the given timeframe.
Recognizing these challenges is key; it helps data analysts adopt proactive strategies to mitigate obstacles. This enhances the effectiveness and reliability of insights derived from a data analytics case study.
Now, let’s talk about the best software tools you should use when working with case studies.
Top 5 Software Tools for Case Studies
In the realm of case studies within data analytics, leveraging the right software tools is essential.
Here are some top-notch options:
Tableau: Renowned for its data visualization prowess, Tableau transforms raw data into interactive, visually compelling representations, ideal for presenting insights within a case study.
Python and R Libraries: These flexible programming languages provide many tools for handling data, doing statistics, and working with machine learning, meeting various needs in case studies.
Microsoft Excel: A staple tool for data analytics, Excel provides a user-friendly interface for basic analytics, making it useful for initial data exploration in a case study.
SQL Databases: Structured Query Language (SQL) databases assist in managing and querying large datasets, essential for organizing case study data effectively.
Statistical Software (e.g., SPSS, SAS): Specialized statistical software enables in-depth statistical analysis, aiding in deriving precise insights from case study data.
Choosing the best mix of these tools, tailored to each case study’s needs, greatly boosts analytical abilities and results in data analytics.
Final Thoughts
Case studies in data analytics are helpful guides. They give real-world insights, improve skills, and show how data-driven decisions work.
Using case studies helps analysts learn, be creative, and make essential decisions confidently in their data work.
Check out our latest clip below to further your learning!
Frequently Asked Questions
What are the key steps to analyzing a data analytics case study?
When analyzing a case study, you should follow these steps:
Clarify the problem: Ensure you thoroughly understand the problem statement and the scope of the analysis.
Make assumptions: Define your assumptions to establish a feasible framework for analyzing the case.
Gather context: Acquire relevant information and context to support your analysis.
Analyze the data: Perform calculations, create visualizations, and conduct statistical analysis on the data.
Provide insights: Draw conclusions and develop actionable insights based on your analysis.
How can you effectively interpret results during a data scientist case study job interview?
During your next data science interview, interpret case study results succinctly and clearly. Utilize visual aids and numerical data to bolster your explanations, ensuring comprehension.
Frame the results in an audience-friendly manner, emphasizing relevance. Concentrate on deriving insights and actionable steps from the outcomes.
How do you showcase your data analyst skills in a project?
To demonstrate your skills effectively, consider these essential steps. Begin by selecting a problem that allows you to exhibit your capacity to handle real-world challenges through analysis.
Methodically document each phase, encompassing data cleaning, visualization, statistical analysis, and the interpretation of findings.
Utilize descriptive analysis techniques and effectively communicate your insights using clear visual aids and straightforward language. Ensure your project code is well-structured, with detailed comments and documentation, showcasing your proficiency in handling data in an organized manner.
Lastly, emphasize your expertise in SQL queries, programming languages, and various analytics tools throughout the project. These steps collectively highlight your competence and proficiency as a skilled data analyst, demonstrating your capabilities within the project.
Can you provide an example of a successful data analytics project using key metrics?
A prime illustration is utilizing analytics in healthcare to forecast hospital readmissions. Analysts leverage electronic health records, patient demographics, and clinical data to identify high-risk individuals.
Implementing preventive measures based on these key metrics helps curtail readmission rates, enhancing patient outcomes and cutting healthcare expenses.
This demonstrates how data analytics, driven by metrics, effectively tackles real-world challenges, yielding impactful solutions.
Why would a company invest in data analytics?
Companies invest in data analytics to gain valuable insights, enabling informed decision-making and strategic planning. This investment helps optimize operations, understand customer behavior, and stay competitive in their industry.
Ultimately, leveraging data analytics empowers companies to make smarter, data-driven choices, leading to enhanced efficiency, innovation, and growth.